Increasing the concurrency of a Kafka consumer 🧮
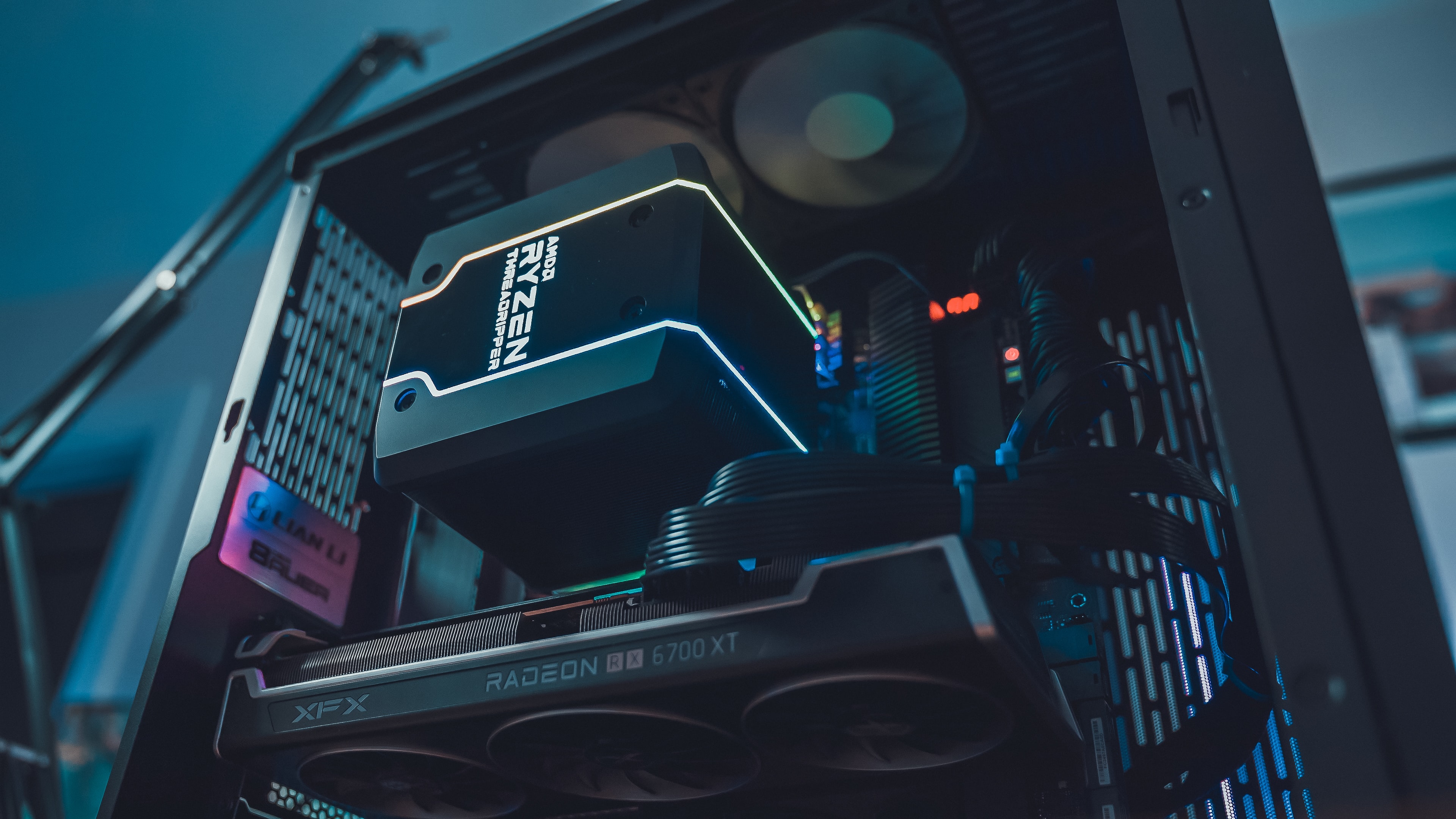
Multithreading is “the ability of a central processing unit (
CPU
) to provide multiple threads of execution concurrently, supported by the operating system.”
Imagine you have multiple producers publishing to a kafka topic.
Now, let’s consider the consumer(s) of this topic.
By default, kafka reads and processes messages in a single-threaded fashion.
It uses a poll()
operation to fetch data records from a topic.
Generally, this operation is smooth because it is preferable that the processing we do between each poll is fast and efficient.
If this processing becomes inefficient… well then, a few nasty things happen ☠️
The code used in this blog can be found here 🤌
Kafka rebalancing⌗
When a consumer polls and tries to fetch data from a topic, it will also send a heartbeat 💓 to kafka. This is signalling to the cluster that it is in a healthy state, thereby extending its lease on consuming from the topic’s partitions.
If the length of time between heartbeats is too long (> session timeout period), then kafka will assume that this consumer is dead and will trigger a rebalance.
Explaining what happens under the hood during a rebalance is beyond the scope of this blog, but keeping it short, a rebalance occurs whenever the number of consumers in a consumer group changes ( for e.g. when a new consumer joins a consumer group, or when a consumer is marked as dead).
During this operation, Kafka will redistribute the partitions among healthy members of the group and all consumer instances (in the consumer group) will stop reading from partitions and committing any pending offsets. The time taken for a rebalance can be significant too.
So as you can imagine, constant rebalances can be problematic… they are essentially periods where we do not process any data!
What can we do about this?⌗
1️⃣ Increase
max.poll.interval.ms
The most common cause of rebalances is that the processing times for some applications take too long 😴 … it exceeds max.poll.interval.ms
and therefore triggers a rebalance.
Simple solution is to increase this value.
⚠️This can come with some side effects. Kafka will only revoke partitions when a consumer finishes its current poll. If the processing within this poll() takes hours, then you got it, rebalancing will take hours too.
2️⃣ Improve processing performance by scaling up the number of consumers
The main way we scale data consumption in kafka is by adding more consumers to a consumer group.
The maximum number of consumers in a group is bound by the number of partitions in a topic. Extra consumers will just remain idle since all the partitions have been distributed among consumers.
poll()
processing times can be decreased by reducing the batch size. For e.g. instead of a single subscriber consuming/processing a batch of 100 messages in one poll()
call, we can have 10 subscribers consuming 20 messages. This will decrease poll times whilst also increasing throughput.
⚠️ Solving slow processing by increasing the number of partitions/consumers = throwing 💵 at the problem.
Multi-threaded consumer model⌗
In this blog, we will explore ways to increase the concurrency of a kafka consumer so that we can achieve more with a single consumer than simply increasing the number of partitions.
We will explore a multi-threaded consumer model.
The jist of this approach is as follows:
- A single main thread will call
poll()
and fetch data from multiple / single partition(s). - Once a batch of messages has been received by the main thread, we will deliver the messages to a pool of threads where the processing will be done in parallel.
- This pool of threads will be of
length=batch size
(if we have a batch of 20 messages, the total number of threads used 20) - Offset management becomes paramount in this approach
If we allowed child threads to commit offsets, this can lead to synchronisation issues (🏎️ conditions) and data loss or data duplication.
- Therefore, we will ensure that the main thread is the only thread that commits an offset. It will wait for all threads to finish processing. This is to ensure that all messages are processed and their offsets are committed in a coordinated and synchronous manner.
So without further ado, let’s get coding 🧑💻 …
The code⌗
First things first, we need a kafka cluster up and running.
All of the processes used in this experiment will be containerised!
Let’s create a simple docker-compose.yml
file that will do all this magic for us 🧙♂️
To build or pull the docker images and create the services, run:
This should create 6 running services:
The kafka dashboard can be accessed by going to http://localhost:8080.
ELI5: What’s happening?⌗
The producer will publish a JSON payload to a kafka topic for some set duration.
The payload will look like:
Upon receipt of this message, the consumer will sleep for the value specified in the payload.
The publish rate will be a measly ~
360msgs/minute
.
Threading disabled ❌:
With threading disabled, the consumer will pull down messages in batches of 20 and for each message, a sleep command will be executed. This will be done in a sequential fashion by the main thread:
After the batch has been processed, the last offset of the batch will be committed by the main thread. This is letting kafka know that this batch has been processed successfully.
Threading enabled 🟢:
With threading enabled, the sleep command will be handed off to multiple child threads for execution.
For e.g. consider the consumer pulling down a batch of 20 messages. Each sleep command will be executed concurrently within the process via child threads:
The main thread would wait for all threads to finish executing before committing the last offset of the batch to kafka.
It’s possible to monitor the number of threads the python container is spawning by running:
Now, let’s delve into the python logic 🕵️…
The producer⌗
👇
In a nutshell:
- The code creates a Kafka producer which sends data to a Kafka broker.
- It uses the
confluent_kafka
library to interact with the broker.- The publish interval,broker address and topic are determined by the environment variables:
PUBLISH DURATION
,KAFKA_BROKER
andKAFKA_TOPIC
with default values60
,localhost:9092
andtest
, respectively- These env vars are set inside
docker-compose.yml
(You can also include these in a.env
var)- The
produce_loop
function generates a JSON payload which will be published to the given topic 5 times every second. This function will continue to run until the publish interval has elapsed.- The
delivery_report
function is a callback that logs a message upon successfuly delivery or logs an error if delivery fials.
The consumer⌗
👇
In a nutshell:
- The
consume_messages
function creates a consumer instance and subscribes to a specified topic.- It uses a while loop to continuously poll for messages(
long-lived consumer
), and processes messages in batches.- If multi-threading is enabled, the function starts a new thread for each message in the batch, and each thread processes a single message.
- If multi-threading is disabled, the function processes all messages in the batch sequentially in the same thread.
- After processing the messages in a batch, the
main thread
commits the offsets of the processed messages.- The
process_msg
function is used to process individual messages. It will extract a sleep time from the message, and sleeps for the specified sleep time.
The results⌗
Using a single-threaded approach, all messages were processed in ~12 minutes, with each batch taking approximately: 39.8s
😅
Using a multi-threaded approach, all messages were processed ~ 55 seconds, with each batch taking approximately: 3.03s
🔥
What’s better and more important than the improvement in performance?
The committal of the offset was synchronised and performed by the main thread 🔐
Pretty good, eh? 🚀